In the era of data, referred to as the “new gold” of the business, not just resources, but also is a key factor determining competitiveness in the market. However, with the explosion of big data, the extraction of value from data is not easy. This is the time Data Mining – a method of data analysis modern – become important tools for businesses to find out the valuable information hidden from that support strategic decisions and optimize business processes.
This article Lac Viet Computing will help you understand Data Mining what isrole of it in business, the engineering, data mining, common, the same challenges, and specific solutions when deployed business help you take maximum advantage of the power of data to reach on the development process.
1. Data Mining what is?
Data Mining (data mining) is the process to discover, analyze and extract useful information from large data sets. Through the use of tools, techniques such as statistics, machine learning (machine learning), artificial intelligence (AI), data mining helps to identify patterns, trends or relationships implicit in the data that previously was not easy to get out.
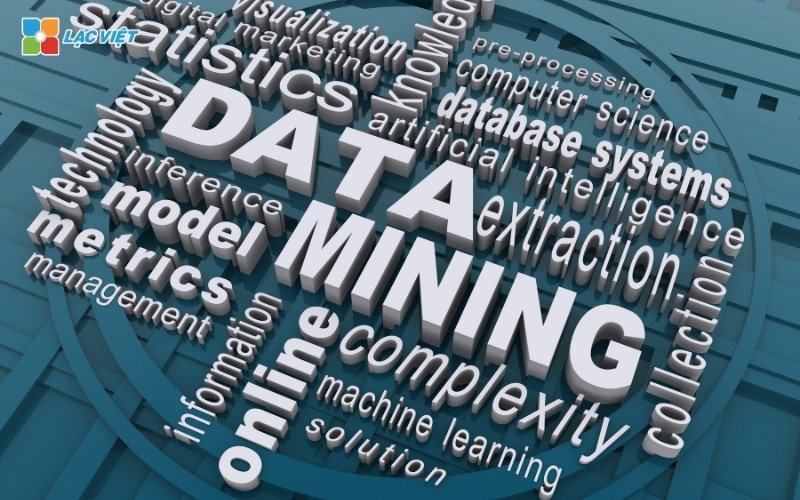
The origins of Data Mining derived from areas such as:
- Statistics: Used for the analysis and data modeling.
- Artificial intelligence: Use smart algorithms to process and learn from data.
- Management system database: Store and manage large data sets, support information extraction efficiency.
Illustrative examples: Imagine a retail business, there are data on the buying behavior of customers in the past 5 years. Through data mining, businesses can detect that customers often buy products A and B together, from which deploy campaigns, cross-selling (cross-selling) efficiency.
2. Benefits of Data Mining for business
Evidence practices showed the important role of Data mining in business
According to Gartner, the business applying Data Mining in business processes has increased performance by 25%. This is thanks to the ability to detect quickly the opportunities and risks in the data, to help businesses react in time before the fluctuations of the market.
Data from McKinsey: A study by McKinsey indicates that:
- 80% of businesses apply Data Mining has significantly improved the ability to predict the market.
- 73% of business leaders believe that data mining help them make strategic decisions better, particularly in the forecast customer demand and optimize costs.
2.1 minimize business risks by analyzing historical data
Data mining helps businesses use historical data to analyze patterns and trends. Through it, businesses can detect potential risks in business activities such as drop in revenue, changes in customer behavior, or supply chain interruption.
For example, A bank using Data Mining to analyze the credit history of the customer. The results showed that a group of customers tend to delay payments when interest rates exceed a certain threshold. Bank from that adjustment lending policies to minimize the risk of bad debts.
2.2 Forecast consumer trends and market changes
With the ability to analyze real-time data, Data Mining help businesses predict consumer trends in the future. This is extremely important in the industry competitive as retail, technology, real estate.
For example, A company, e-commerce, data analysis, shopping in the occasion to forecast consumer trends. The results help them increase reserves-selling products, reduce inventory not needed, optimize revenue.
Data mining provides the overview angle support business to predict the future trends. For example:
- Banking sector: Predicting credit risk, based on transaction history.
- Manufacturing industry: Detect potential problems in the supply chain to improve operational efficiency.
- E-commerce: Hint products in accordance with customer preferences.
2.3 analysis of behavior, customer preferences
Data Mining provides the enterprise with a holistic view of customer behavior, including the products they usually buy time they shop, factors influencing purchasing decisions.
For example: A store online retailers use Data Mining to detect that the customer usually add products to cart in the evening, but complete the transaction in the morning. Thanks to that store, implement campaigns reminders via email in the morning to increase the percentage of completed orders.
2.4 personalize the shopping experience, increase satisfaction and customer retention
Through the mining of personal data and transaction history, businesses can personalize each customer experience, such as product suggestions fit, send notifications promotion right time or to provide after-sales service better.
For example: Netflix uses Data Mining to analyze the movie-watching habits of users, from which hints of movies and series in accordance with individual preferences, helps to increase the user experience but also help Netflix to sustain high rates of customer retention at a high level, up to 25%.
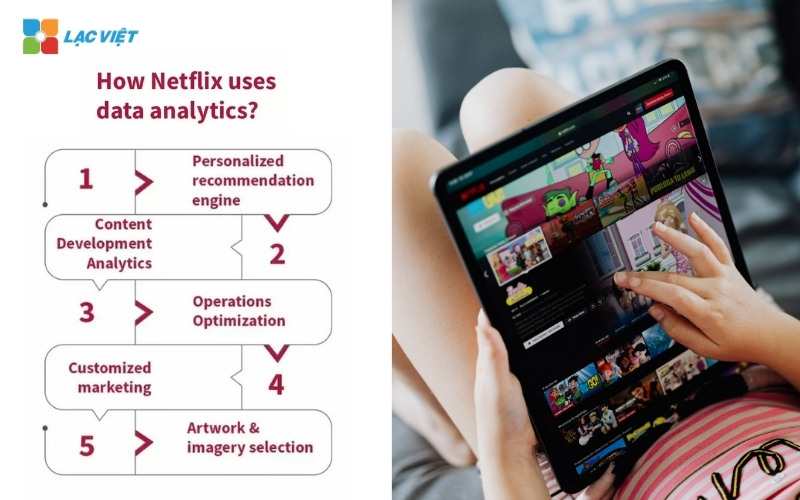
2.5 detection of points of congestion in the supply chain and optimize operating
Data mining helps businesses track the whole process from production to distribution, to detect points of congestion in the supply chain. This does not only reduce operating costs but also ensure transparency in business operations.
For example, A logistics company, and apply Data Mining to data analysis, shipping, thereby realize that the order in the city was delayed due to the delivery route is not optimal. After adjustment for route delivery time, reduced by 15%.
2.6 application in risk management and financial fraud
Support detection of abnormal behavior in finance, such as fraudulent transactions or activities not valid.
For example, A credit card companies use Data Mining to detect unusual transactions, such as buying high value items in different locations in a short period of time. Automated system alerts and pause the transaction to verify with the customer.
3. The technical methods, data mining, popular
3.1. Classification (Classification)
Classification (Classification) is a technique of data mining is used to assign objects in the data set into groups (class) specific based on attributes already know. This is a prediction method, to help businesses that rely on historical data to determine the properties of new objects.
Practical application:
- Bank: Classify customers into groups of “high risk,” “low risk” to assess the likelihood of credit.
- E-commerce: Classify customers by age, behavior, shopping area, to personalize marketing strategy.
Benefits:
- Enhance the accuracy in predicting customer behavior.
- Enhance effective decision-making, thanks to understand the target group.
3.2. Clustering (Clustering)
Clustering (Clustering) is the technical group of objects in the data into the cluster (cluster), a star for the objects in the same cluster have the same characteristics and differences with other clusters.
Practical application:
- Marketing: Clustering customers based on shopping behavior to optimize ad strategy.
- Health: Clustering patients based on symptoms to determine the method of treatment is suitable.
Practical examples: A retail company that uses clustering to group customers according to the shopping habits: “the regular shopping”, “people shopping in holiday”, “the only purchase discount.” The results help companies optimize marketing campaigns for each group.
Benefits:
- Detection segment of the market potential.
- Support personalization business strategy.
3.3. Regression (Regression)
Regression (Regression) is a technique of Data Mining is used to predict the value (continuous value) based on the relationship between the variables.
Practical application:
- Revenue forecast: Predict revenue based on factors such as trend and shopping advertising budget.
- Finance: Analyze market data to forecast stock prices.
Benefits:
- Providing accurate predictions to support planning business.
- To help businesses understand the relationship between the factors that affect business results.
3.4. Exploring the relationship (Association Rule Mining)
Exploring the relationship is a technical Data Mining helps to detect sample relationship potential between the data items in large data sets.
Practical application:
- Retail: Detect the products are often purchased together (e.g., diaper, child, and beer).
- E-commerce: Product recommendations based on purchase history.
Practical examples: A supermarket chain used to explore the relationship to detect that customers usually buy bread with peanut butter. Since then, they deploy the promotion “buy bread discounts peanut butter,” increased sales by 20%.
Benefits:
- Increase sales through cross-selling (cross-selling).
- Improve shopping experience by suggesting the right products.
3.5. Time series analysis (Time Series Analysis)
Time series analysis what is?
Time series analysis is the technique data mining to forecast trends based on data collected over time.
Practical application:
- Financial planning: Predict sales according to the season.
- Supply chain: Analysis needs to optimize inventory.
Practical examples:
A fashion companies use time series analysis to predict demand for winter clothing to help increase the accuracy in the production and reduce the amount of excess inventory.
Benefits:
- Help business plan more effective.
- Accurate predictions of market trends in the future.
4. The software tool supports data mining
4.1 RapidMiner: visualization tool and data analysis strong
RapidMiner is one of the tools data mining today's top is designed to support users at all skill levels, from beginners to experts of the analytical data.
Feature highlights:
- Interface drag-and-drop, easy to use without the need to write code.
- Integrated learning algorithm machine (machine learning) to analyze and predict the data.
- Ability to handle big data with fast speed.
Practical application: A company's e-commerce use RapidMiner to analyze buying behavior and predict product demand, thereby increasing sales up 20%.
4.2 WEKA: Support data mining with multiple algorithms, machine learning integration
WEKA is an open source software popular in the field of Data Mining with multiple algorithms, machine learning, powerful for professionals to analyze data.
Feature highlights:
- Support diverse algorithms such as clustering (clustering), sorting (classification), regression (regression).
- User interface simple, easy to learn and use.
- The ability to integrate with programming languages such as Python or Java.
Practical application: WEKA is used in medical field to analyze patient records, support, doctor, given the more accurate diagnosis.
4.3 Python and R: a programming language popular for data analysis
Python and R are the two programming languages are the most popular in the community data science thanks to the ability to customize high ecosystem rich library.
Python:
- Popular visualization libraries: Pandas, NumPy, Scikit-learn, TensorFlow.
- Pros: versatile, easy to learn, can be used both for data analysis, basic and complex.
R:
- Library downloads: ggplot2, caret, dplyr.
- Advantages: Specialized in statistical analysis, data visualizations.
Practical application: Financial companies often use Python and R to build models to predict stock prices or analysis of credit risk.
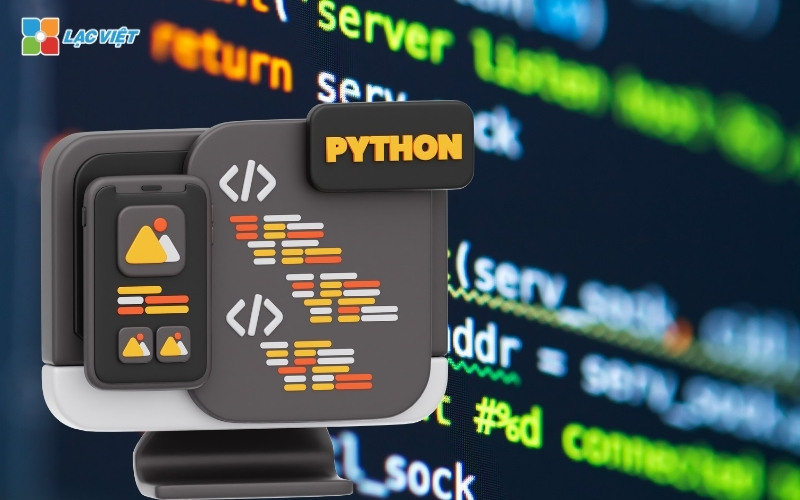
4.4 Tableau and Power BI: Integrated, data mining, visualization results
Tableau and Power BI are the two top tools of data visualizations to help businesses transform the complex numbers into the chart control panel is easy to understand.
Tableau:
- Strong ability to connect multi-source data, create a dashboard to visually.
- Suitable for the data presentation in the meeting.
Power BI:
- Tightly integrated with the Microsoft ecosystem.
- The ability to analyze real-time data and automatically generate reports.
Practical application: A manufacturing company uses Power BI to monitor production performance, detection of bottlenecks, process optimization, help increase productivity up to 15%.
4.5 Google Cloud AI and AWS AI: Support analysis processing big data with AI technology
Google Cloud AI and AWS AI solutions provider, data processing, powerful, integrated technology artificial intelligence to automate the analysis process, the predicted data.
- Google Cloud AI: Service AutoML helps to build the machine learning models without the need for in-depth knowledge about programming.
- AWS AI: Support tools such as Amazon SageMaker to train and deploy machine learning models quickly.
Practical application: A large bank use AWS AI to detect fraudulent transactions, reduce financial losses at 20% in the first year of deployment.
5. Steps to implement Data Mining in business
Step 1. Identify business goals
Clarify goal: Goals should be specific and associated with business needs, for example:
- Predict customer behavior in order to increase conversion rates.
- Optimized production processes to reduce operating costs.
Practical examples: An insurance company set the goal to minimize fraud by analyzing the sample transaction is not normal in the history of the claim.
Step 2. Collect and clean data
Collect data: Use tools like Google BigQuery, AWS, or the CRM system to collect data from multiple sources.
Data cleaning: Remove duplicate data, outdated and not valid to ensure the quality of the input.
Step 3. Choose the method of data mining
Choosing the right method:
Based on the business objectives, the business can choose the method as:
- Cluster (Clustering) to find potential customers.
- Regression (Regression) to predict the trend in sales.
Step 4. Analysis and deployment
Apply algorithm:
Using machine learning algorithms like Decision Trees, Random Forest or Neural Networks to analyze data.
Interpretation of the results:
The results are presented in the chart and dashboard for easy decision-making.
Step 5. Evaluation and optimization
Efficiency rating: Use indicators KPIs to measure the level of success, for example, revenue growth, reduce operating costs.
Continuous optimization: Update the new data and adjust pattern to maintain the long-lasting effect.
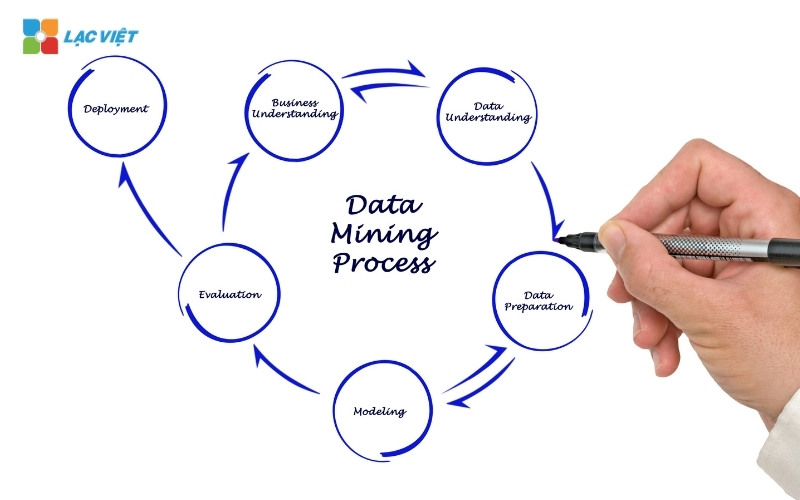
6. Challenge when the application data mining in business
6.1 Lack of staff expertise
One of the biggest barriers when implementing Data Mining is the shortage of personnel with expertise in the field of data analysis. Businesses often have difficulty in:
- Looking for specialists, data analysis, Data Scientist, or Data Engineer experienced.
- Training staff internally to understand, use tools Data Mining.
- Connect departments to leverage data in a synchronized and effective.
A survey from Gartner (2023) indicates that 63% of business said their lack of hr expertise to successfully implement the project, data mining.
6.2 Data heterogeneity or poor quality
Data is the core asset of Data Mining, but not always business also have high-quality data. Common problems include:
- The data is duplicate, outdated, or incomplete.
- Data from multiple sources of heterogeneity (CRM, ERP, social networking, sensors, IoT).
- No data is organization for standardization leads to difficulties in processing and analysis.
Consequences:
- Pattern analysis is not accurate, leading to wrong decisions.
- Time-consuming, the cost to clean, standardized data.
Quote: Follow IBM (2022), data of poor quality, causing damage of up to 3,1 trillion USD per year for the global business.
6.3 security issues and data privacy
The collected data analysis in the digital age faced with the challenge of security of privacy. Common problems include:
- Data leakage: Network attack or manage loose lead to sensitive data being divulged.
- Violation of privacy: The collection and use personal data does not comply with the legal regulations, such as GDPR in Europe or Decree 13/2023/ND-CP in Vietnam.
- Lack of a mechanism to protect data: Business measures are not taken, encryption, data protection, powerful.
Quote:
- A study from Ponemon Institute (2023) said, 45% business join security incident data within the first 2 years of deployment of Data Mining.
- The violation of privacy not only lose credibility but also lead to fines, extreme, making serious impact on business operations.
7. Solution fix
7.1 internal Training and hire expert data
To solve staffing problems, the business can perform:
- Internal training: Organization of the course, data analysis, basic to advanced for employees. Use the tool easy to learn as Tableau or Power BI to build background knowledge solid.
- Hire professionals: Looking for the expert Data Scientist or cooperate with company professional advice to support the deployment, the original guide.
- Build the team in charge of: Establishment division specializes in data, combining expert on information technology, statistics, business analysis.
7.2 investments in technology infrastructure and security tools data
To solve the problem of quality and data security, businesses need to:
Technology infrastructure:
- Construction, storage systems, data management concentration (Data Warehouse).
- Use cloud platforms like Google Cloud or AWS ability to expand storage, data processing.
Security tools:
- Deploy the solution data encryption (encryption) to protect sensitive information.
- Use the system detect and prevent network attacks, such as SIEM (Security Information and Event Management).
Compliance with laws:
- Build process collects data processing complying with the legal regulations such as GDPR or CCPA.
- Staff training about the privacy policy and the privacy.
7.3 process optimization, clean, standardized data
To ensure input data are of high quality, business need:
- Use specialized tools: Apply tools like Trifacta or OpenRefine to automatically clean and standardized data.
- Construction standard procedures: Rule definitions, clear standards for collecting data storage.
- Check data periodically: Perform the quality check the data regularly to detect and handle problems soon..
Data Mining not only is a tool, which is the bridge to help businesses explore and transform data into a competitive advantage. From the analysis of customer behavior, forecasts, market trends, to optimize operation, data mining, brings the practical value, help in business decisions strategy correctly and efficiently.
Though the process of deployment Data Mining can face many challenges, but with thorough preparation, investing in technology and building a team of the quality, businesses can fully overcome the barriers to leveraging the maximum potential of data. Let's start the journey of data mining today, so the data is not just numbers that became the impetus take your business far more than the market competition is fierce!