According to McKinsey (2023), the organization applies the method to Data Driven increase of 23% business performance than organizations using traditional methods. However, neither organization would also know how to harness maximum power from the data to take strategic decisions and optimize business processes. This is the time method Data Driven – decisions based on data – become the inevitable trend for the modern enterprise.
This article Lac Viet Computing will help you to understand the concept of Data Driven reasons why businesses should apply this method step by step deployment efficiency to maximize value from data, from which to create breakthrough in business activity.
1. The popularity of big data (Big Data) in business
Big data (Big Data) are increasingly becoming the platform in making business decisions. The exponential increase of connected devices, IoT (Internet of Things), online transactions, social networking has created a mass of huge data. According to a report from IDC (2023), the world will create more than 175 zettabyte data in 2025, the business would take advantage of this data will have big advantage in the innovation development.
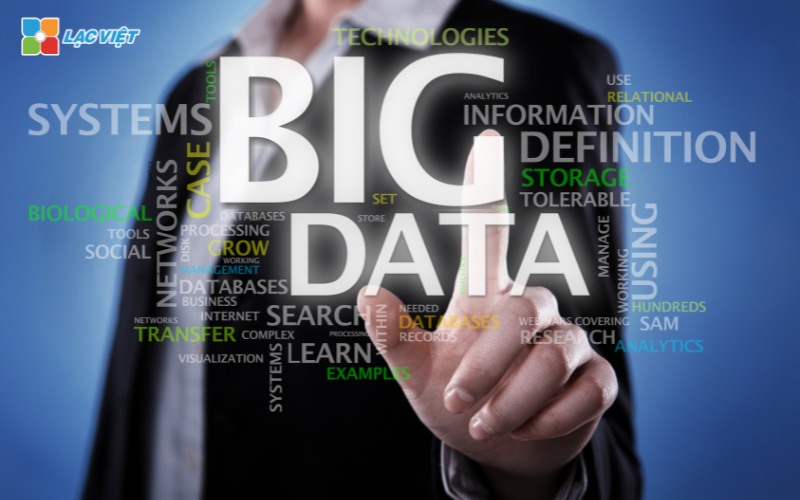
For example:
- The company e-commerce: Use Big Data to analyze the shopping behavior of the customers, which product suggestions accordingly.
- Logistics: Analyze real-time data to optimize supply chain.
Report from Gartner about the value of data according to the study of Gartner (2023):
- 87% of business for that data is the core asset of them.
- 66% of business has significantly increased spending on data analysis within the past 3 years.
This proves that the focus on data is not only a trend but also the long-term strategy of the modern enterprise.
Let's start the journey Data Driven right from today to take your business to progress further!
2. Data Driven is what?
2.1. Introduce the concept of “Data Driven”
Data Drivenor, “based on the data,” is an approach in business, in that the decision was made based on data analysis instead of emotional, personal experience or predict subjective. This approach focuses on the extraction of value from data through tools and modern technology to make decisions accurately and quickly, there are clear grounds.
For example, Instead of predicting the market demand based on experience, a business Data Driven will analyze sales data, customer behavior and market factors to forecast the trend correctly.
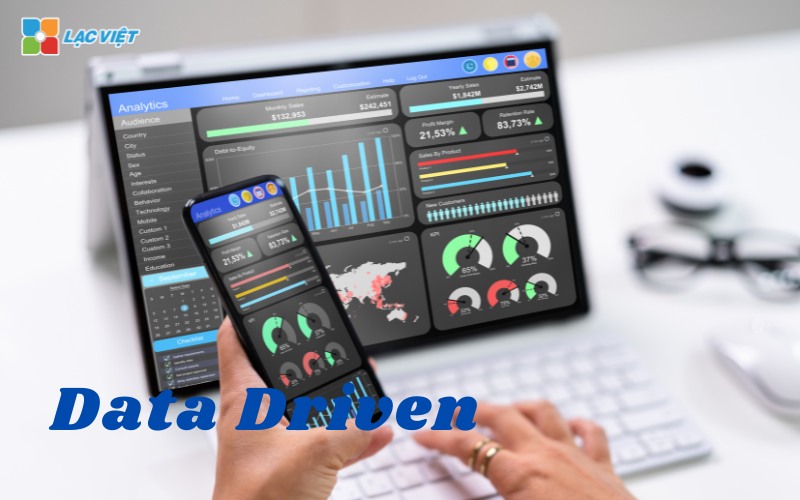
2.2 importance in the conversion of
In the context of transformation of the current data, referred to as the “oil” of the 21st century. Businesses use data not only to react to changes, but also predict trends, optimize processes and create competitive advantage. Data Driven gives businesses the ability to:
- Understand more customers: Behavior analysis, demand and consumption habits to personalize the experience.
- Improve operational efficiency: Automate and optimize business processes based on real data.
- Take the correct decision: Remove emotional in business decisions, help businesses predict risks and opportunities.
Business Data Driven not only the trend but also the factors that determine success in the environment of modern business. The difference between a business lead and a business according to the following usually located in the business that can effectively leverage their data or not.
3. Why businesses should adopt Data Driven?
3.1 decision-making more accurate
The data provide accurate information, thus helping the business make decisions based on fact rather than emotion.
- Practical examples: A supermarket chain in the U.s. using data analytics to forecast demand goods according to each season, helping to reduce excess inventory to 25%.
- Statistics: Harvard Business Review said business apply decisions based on data, have the ability to achieve high efficiency over 19% compared with the competition.
3.2 Increase operational efficiency
Data Driven help automate operational processes, thereby saving time and resources.
- For example: Use data analysis to optimize production schedules, reduce wasted time and materials.
- Fact: A study of McKinsey (2022) indicate that the optimized process operation data helps to reduce operational costs up to 15-20%.
3.3 Understand customer behavior
Businesses use data to analyze the behavior, preferences and needs of customers, from that given strategy personalization experience.
- Typical example: Netflix data usage view movie of users to suggest appropriate content, increase the rate of customer retention by 25%.
- Results: A report of Salesforce shows 70% of customers expect brands to understand their needs through data.
3.4 Increase competitiveness
Business operation based on data always has more advantages in the rapid response before the change of market and customer needs.
- For example: Amazon uses data analysis to manage inventory and optimize prices in real time, create competitive advantage superior to the opponent.
- Quote: Report from Deloitte (2023) said 56% of businesses apply Data Driven achieved revenue growth exceeds expectations.
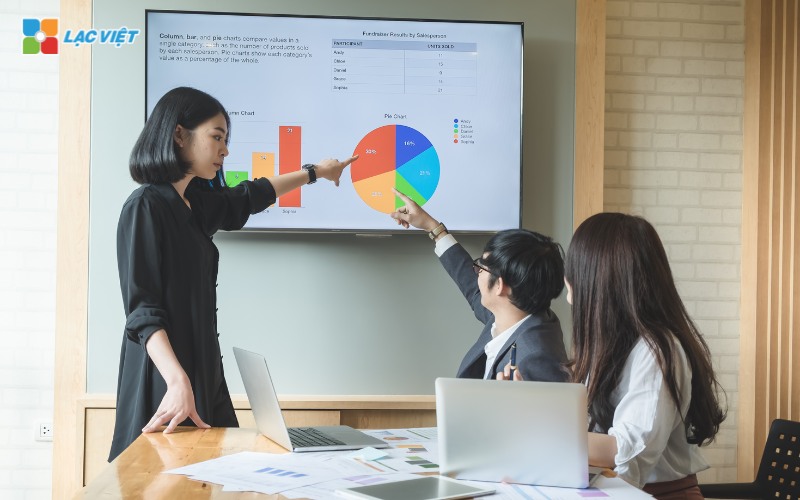
4. Steps to build business Data Driven
4.1. Building a culture of data in the organization
Why the need for cultural data?
Building a culture of data is the first step and the most important to the business to become Data Driven. Cultural data not only is the tool or software that also requires a change in thinking and way of working of the whole organization.
The core elements:
- Commitment from leadership: Leaders need to aware of the value of the data and lead the organization to apply the approach based on data.
- Promote school spirit asked: Training staff on how to use data encouraged to use data in their daily work.
- Transparent and share: Build storage system and data access transparency, which allows departments to easily share and use data.
According to the report of Deloitte (2023), 73% of organizations succeed with Data Driven start by changing the culture data.
4.2. Define your business goals clearly
Why clear objectives important?
A strategic Data Driven efficiency should be built based on the specific goals, measurable and closely linked with the business strategy.
How to define the target:
- SMART Framework: Goals should be specific (Specific), measurable (Measurable), feasible (Achievable), realistic (Realistic), there are time limits (Time-bound).
- Link to business results: All target Data Driven to support the business to achieve business efficiency, such as increasing revenue, reducing costs or improving customer experience.
For example: A company's e-commerce can set goals as follows: to Increase the rate of customer conversion from 2% to 4% in the next 6 months using data analysis customer behavior.
4.3. Collect and clean data
Why clean data important?
Data inaccuracies or omissions will lead to the wrong decision. Therefore, the process of obtaining and cleaning data are key steps to ensure data quality.
How to perform:
- Collect data: Use modern tools to automate the process of collecting data from multiple sources such as CRM, ERP, social networking platforms, e-commerce.
- Data cleaning: Remove duplicate data, outdated, or incorrect. The steps include standardized format, to verify the validity, fill the missing data.
Tool support:
- Google BigQuery: Processing and analyzing big data.
- Tableau: Integrate data from multiple sources and create visual reports.
- Power BI: Create the report, comprehensive analysis.
4.4. Analysis and visualization of data
Why need to analyze and visualize data?
Data analysis help businesses harness the profound meaning from data, while data visualizations to help convey information in an understandable way, quickly.
Steps taken:
- Data analysis: Use analysis tools to identify trends, predict the results and find business opportunities.
- Visualization: Charts, graphs and dashboard help leaders quickly understand and make a decision.
Popular tools:
- Tableau: Create the report visually appealing.
- Power BI: Provide the chart and dashboard easy to understand.
- Python/R: Support data analysis, enhanced with sophisticated algorithms.
Quote: Follow Forbes Insights (2023), the business applied data analysis visually increase productivity up to 15%.
4.5. Measurement and continuous optimization
Why need to measure and optimize?
Data Driven strategy was not done once that is continuous process. To ensure efficiency, businesses need to regularly measure results and optimize strategies based on new data.
How to measure:
- Define KPIs: The key performance indicators should be directly related to business goals. For example, conversion rate, revenue, or operating costs.
- Periodic analysis: Data tracking weekly, monthly, to evaluate the effectiveness.
Optimization:
- Based on the measurement results, adjust strategies and processes to improve performance.
- Using AI and Machine Learning to detect new trends or potential risks.
For example: A retail company applied analysis, KPIs, weekly and noticed a particular product sales decreased due to not advertised properly. The company has adjusted marketing strategies to increase product sales up 20% after only 2 weeks.
5. Technology tools to support Data Driven
5.1 Google Analytics: Measuring effective marketing strategy
Google Analytics is an analysis tool powerful data, is widely used to measure and evaluate effective marketing campaign. This tool allows businesses to track user behavior on the website, from which detailed analysis of:
- Traffic sources: Understand the customers come from and where (SEO, advertising, social networking).
- User behavior: Time on page, bounce rate, pages are most visited.
- Conversion: Track the customer journey, from when to visit to time purchase.
Benefits highlights:
- Help optimize marketing campaigns by analyzing real data.
- Make decisions based on trends in customer behavior.
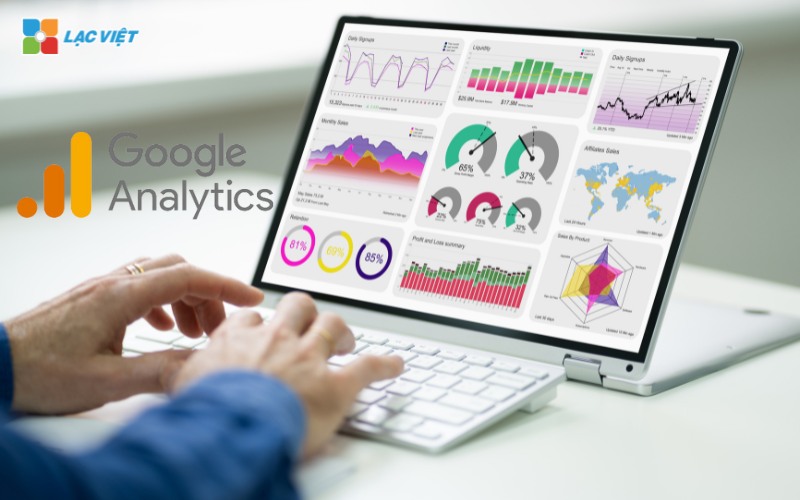
5.2 Tableau: data visualizations
Tableau is the leading tool in the data visualizations, help turn dry numbers khan chart, control panel is easy to understand and lively.
Main features:
- Integrate data from various sources.
- Create charts, graphs, dashboard visually in real-time.
- Supports drag and drop, easy to use even with people who do not specialize in data.
Benefits highlights:
- Increase efficiency analysis thanks to visualize complex data.
- Help leaders rapidly capture information and decision-making.
5.3 Power BI: synthesis and analysis of comprehensive data
Power BI is the tool of Microsoft that is designed to aggregate, analyze data from many different sources, provides a comprehensive overview for business.
Main features:
- Seamless integration with the Microsoft ecosystem (Excel, Azure).
- Support analysis of complex data with features integrated AI.
- Create reports with the ability to customize high.
Benefits highlights:
- Given the detailed analysis and predict business trends.
- Support management and performance optimization business.
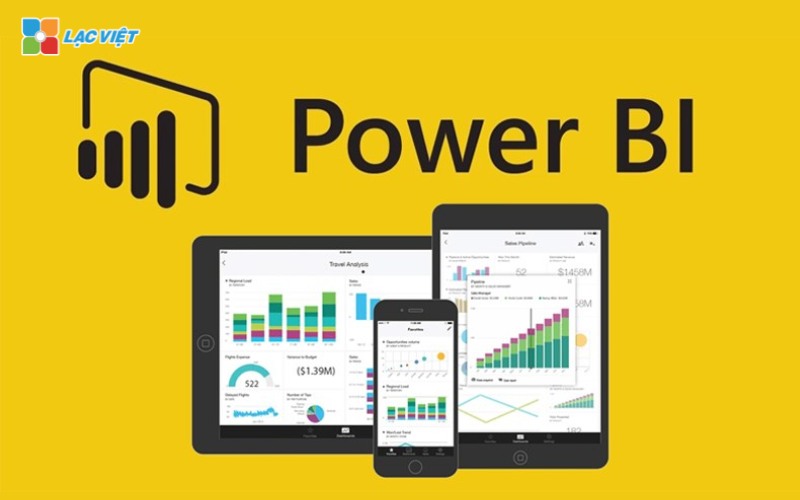
5.4. AI and Machine Learning in Data Driven
Automation big data analytics
AI and Machine Learning played a key role in the processing and analysis of big data (Big Data). The algorithm automatically learns from the data helps to reduce analysis time and increase accuracy.
Specific application:
- Analyze customer behavior: WHO discovered the template shopping behavior and predict future needs.
- Trend prediction: Machine Learning to predict changes in the market based on historical data.
- Process optimization: AI automation task repeated, saving time and resources.
Practical examples: A bank uses AI to detect suspicious transactions, up 25% financial fraud only after 6 months of deployment.
AI predict business trends, future more accurate
WHO doesn't just stop at the analysis, but also help businesses predict the future based on current data. This is especially important in areas such as finance, marketing, supply chain management.
Areas of application:
- Finance: AI predicts stock price fluctuations or credit risks.
- Marketing: WHO determine the market trends and optimize advertising campaigns.
- Supply chain: Machine Learning to predict product demand to optimize inventory.
According to a report from PwC (2023), the business use AI to predict trends, growth, ability to gain higher revenue by 21% compared to business do not apply.
6. Case Studies: successful Business with Data Driven
6.1. Amazon: personalized products based on customer data
How Amazon apply Data Driven:
Amazon uses algorithms Machine Learning to analyze data from the history, shopping, views, reviews of customers. From there, the hint system products that customers are likely to interest most.
Results achieved:
- 35% increase in revenue: The proposed products based on data major contribution to the sales revenue of Amazon.
- Increase customer experience: Personalization products that help retain customers long-term.
Understanding the needs and customer behavior through data to help Amazon maintain its leading position in the market of e-commerce.
6.2. Netflix: optimizing the user experience by analyzing the data
How Netflix apply Data Driven:
Netflix uses user data, including history, watch movies, time stop, ratings of customers, to build the proposed algorithm content.
Results achieved:
- The rate of customer retention increased by 25%: Thanks to the proposed film accurate, and in accordance with the preferences viewers.
- Effective content higher: Netflix data analytics to decide production movies or series based on the popular hobby of the user.
Typical example: Popular Series House of Cards is produced after Netflix noticed the huge demand from viewers about the kind of politics based on data analysis.
7. Challenges when deploying Data Driven
7.1. Skills data analysis in the organization
Challenge: One of the biggest barriers for enterprises to deploy Data Driven is the lack of staff have skills data analysis. Many businesses have difficulty in:
- Understand and harness the data properly.
- Use the analytical tools such as Tableau, Power BI, or Python.
- Interpret data to make business decisions accurately.
Causes:
- Lack of training on data analytics in the organization.
- Employees are often afraid to change or are not yet familiar with the use of new technological tool.
- No data professionals (Data Scientist) or expert data analysis internally.
Solution:
- Internal training: Held in-depth course on data analysis for employees.
- Hire professionals: Recruitment experts analyze data to guide and develop strategic Data Driven.
- Use simple tools: Start by the tool user-friendly as Google Analytics or Power BI before expanding into solution more complex.
7.2. A matter of security and data privacy
Challenge: When collecting and analyzing data, business faced with problems related to:
- Data leakage: Sensitive data of customers or business from unauthorized access.
- Privacy: Ensure that the collection and use of data in compliance with privacy regulations such as GDPR (Europe), or Decree 13/2023/ND-CP in Vietnam.
Causes:
- Infrastructure security is weak, not enough ability to protect data.
- Lack of understanding of the legal provisions related to data.
- Using the analytical solution does not guarantee safety.
Solution:
- Investing in security: Building systems, data encryption and firewalls to protect data from attacks.
- Ensure compliance with the law: Work with legal professionals to ensure that the process of data processing of the business comply with current regulations.
- Select platform safety: Use tools, data analysis, certified security, such as AWS or Google Cloud.
7.3. The initial investment is high for small and medium enterprises (H3)
Challenge: Small and medium enterprises (SMEs) often have difficulty on the budget when starting to deploy Data Driven. The cost includes:
- Shopping tools and analysis software.
- Hiring or training staff.
- Upgrade technology infrastructure for storing, processing big data.
Causes:
- Small scale should limited budget.
- Not clearly see instant benefits from the investment in data.
Solution:
- Start small: Focus on one or two areas of business to deploy Data Driven before scaling.
- Use cloud solutions: Reduce the cost of infrastructure by using cloud services such as Google Cloud or Azure.
- Find technology partners: Partnered with technology companies to receive support, deploy, and operate.
Data Driven not only is a method that has become one of the deciding factors success in the business environment, competition is fierce today. The application of this method not only helps businesses improve operational efficiency, optimize resources, but also opened up the opportunity to capture the trend, to meet customer needs correctly.