In the era of information explosion, businesses are faced with a data volume is growing, stretching from internal to customer data, and market. So how to medium business advantage is the huge amount of data, just optimized the operation process? Retrieval Augmented Generation (RAG) privacy is the groundbreaking solution to help solve the problem.
In the article below, let's Lac Viet learn RAG what is, how it works and 5 application highlights that business can apply.
1. Retrieval Augmented Generation (RAG) is what?
Retrieval Augmented Generation (RAG) is a method of combining information retrieval and module, student, content to create the natural feedback, accuracy by leveraging huge data warehouse to provide the most relevant information according to the context user.
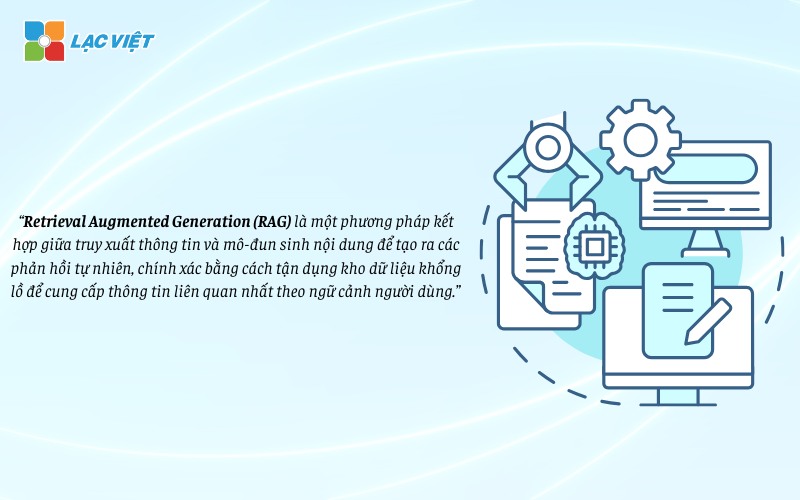
In the context of the business need quick response to the customer request, RAG become effective tools to help optimize interaction between businesses and customers via chatbot system, faq, or the application support service.
Two main components of RAG
- Retrieval Module (module access): This department is responsible for searching, collecting data from the stock available information, such as the internal database, documentation, or online sources. This module ensures that the feedback is created always based on accurate information, as appropriate.
- Generation Module (module a): After the data is retrieved, this module will handle creating feedback using natural language through the language models, big (Large Language Models – LLM). The goal of the module is to create the coherent answer, individualized, user-friendly.
2. Problems of the pattern AI-traditional
The model Generative AI tradition (as ChatGPT), based primarily on data that has been trained earlier, and encounter many limitations when applied in practice:
- Error “Hallucination” (hallucinations information): WHO created the information is not true or not in the training data. For example: When the chatbot was asked about a new product launch recently, it can provide false information or even fabricated information does not exist.
- Misinformation or outdated: ONE based only on the training data from a specific time, unable to update new information if not coach again. For example: A trainer in 2021 could not know about the event or change in policies, business takes place in the year 2024.
- Can't check the source information: Do not cite the source data when the reply, users can hardly verify the correctness of the information. For example: In the field requires transparency as finance or legal, not knowing information is based on sources which is a big risk.
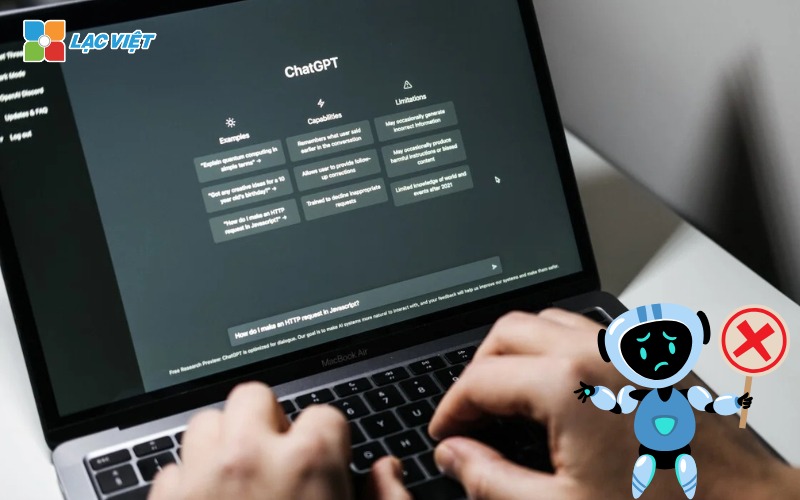
Retrieval Augmented Generation overcome these limitations by combining the ability to access new information, exactly from the data source, external or internal, ensure the answer is not only true but also can be derived.
Examples illustrate the RAG in business
Suppose a business provides services to support customers using chatbot AI. When customers ask:
“Warranty policy of the products X what is the latest?”
- WHO traditional may answer based on old information or give answers general, do not update.
- RAG will retrieve the information from the official database of the company and provide detailed answers, updated: “Product X have warranty policy latest is 12 months, applicable from the date 1/1/2024, including replacement parts for free in case of technical errors.”
3. How the operation of Retrieval Augmented Generation
Retrieval system Augmented Generation (RAG) operate based on strict process consists of 5 main steps:
Step 1: collect data
The process first and most important in the Retrieval Augmented Generation is collecting data, because data is the foundation for the system to operate efficiently. The system retrieves data from various sources, including:
- Source business insider: Guidance documents, technical reports, databases, customer, email, or transaction history. This is the main data system important to help RAG correct answer queries related to internal operations.
- External source: Expert articles, industry research, forum or data publicly online. Data source this ensures the system can provide information that is time, comprehensive.
Calculator updates, main campusc your data is a top priority to ensure the quality of feedback, help businesses always have reliable information to make decisions.
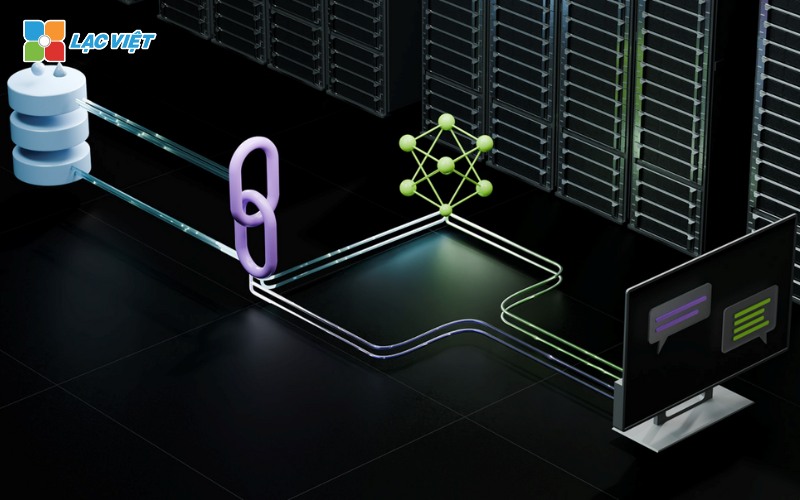
Step 2: divide data
After collection, data is classified, organized in small clusters to increase efficiency in the retrieval.
- Classification mechanism: Based on the factors such as theme, type of informationor features of use. For example, a business service provider, IT can split the data into categories such as “maintenance system”, “security solution” or “technical support”.
- Results achieved: System building a data warehouse structure in which each data item is associated with the keyword or context respectively. This helps to significantly reduce the processing time when the query is sent to.
The division ensures Retrieval system Augmented Generation access information quickly and at the same time limiting the risks to provide information not related.
Step 3: Embed document
Embed documents is an important step to transform text data into a form that the computer can understand and handle. This is done through the algorithm, deep learning (Deep Learning), which helps to convert text into performing the arithmetic (vector embeddings).
- Process operation: The language model as BERT, RoBERTaor Sentence Transformers used in order to analyze the semantics of each piece of text, ensuring data is represented correctly, optimized.
- Effect: Data after embed can be match semantics to the query of the user, regardless of the wording of them. For example, although users asking “how to fix the system?” or “fix IT how?”, system all understand this is the same intent and retrieve the relevant documents.
Thanks to this step, the Retrieval Augmented Generation has the ability to handle complex queries in a smoother way, bring valuable feedback high.
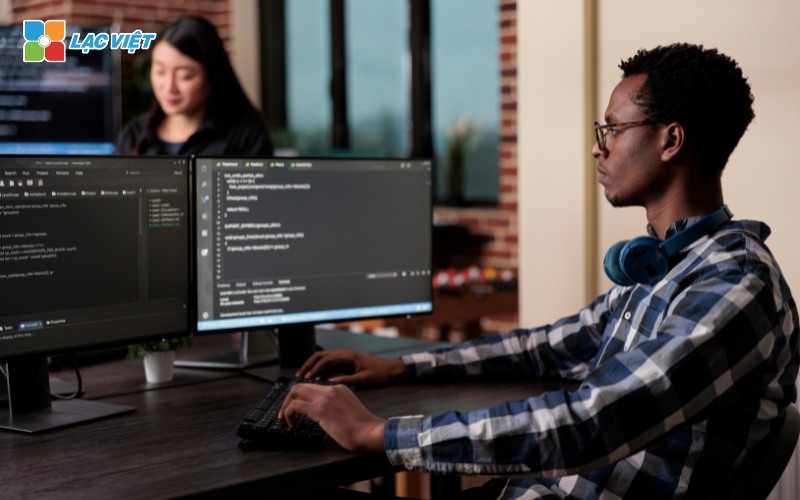
Step 4: Handling user queries
When a user submits a query, the system RAG perform two main tasks: semantic analysis and match information.
- Semantic analysis: Queries are converted into performing vector similar data've embedded, whereby the system to understand the real intention of the user. For example, if the query is “How to make server maintenance efficiency?”, RAG will recognize the keyword “maintenance” and “servers” to search for the matching documents.
- Match information: Based on performances vector, the system searches the data similarities in the repository and returns the most accurate results.
With the ability to handle natural language (Natural Language Processing – NLP), RAG not only answer questions but also provide additional information appropriate to help users have more comprehensive view of the issue.
Step 5: Create feedback with LLM
After retrieval of information, the last step is to use the model language (LLM) to create feedback. Model integrated information access with context query. Then create natural feedback, seamless, consistent with the needs of users.
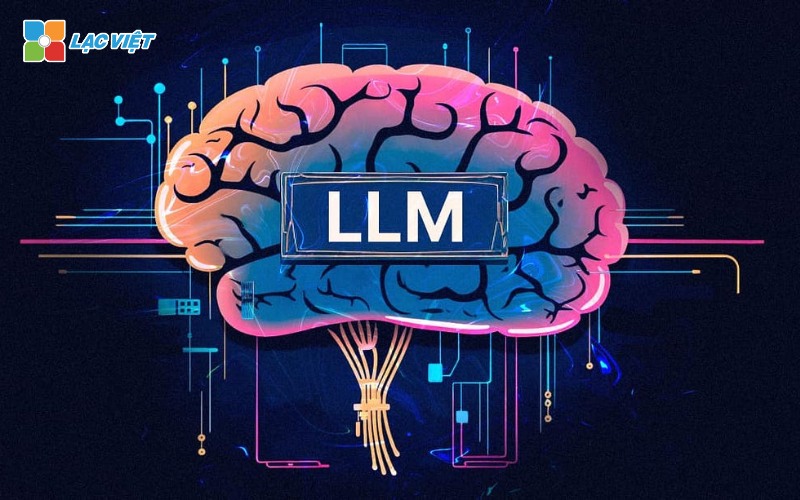
For example, If the user asked, “I need instructions on how to secure intranet system”the response from the Retrieval Augmented Generation can, including procedures for basic security and hints deployment of security tools modern.
LLM provides not only information but also adjust the wording to suit the style of communication of users, create and feel like you are interacting with a real expert.
4. RAG brings the benefits for business?
Retrieval Augmented Generation (RAG) not only is an advanced technology which is also strategic solutions for businesses that want to optimize workflow, enhance performance. Here are the benefits that RAG, help businesses not only overcome the challenges but also prevailed in a competitive environment:
- Increased efficiency in the processing of information: Processing huge amount of data efficiently, thereby improving work productivity. Instead of losing hours and hours to just, sort data, the system automatically retrieve the correct information in just a few seconds.
- Personalize customer experience: RAG doesn't just stop at answering questions, but also analysis of behavior, interests, and status of each customer to give feedback accordingly.
- Analyze and provide timely information: The system can access the reports, market analysis, information about the latest trend or historical data in just a snap. Help the management team make decisions based on real data, there are clear grounds.
- Increased processing speed problems: When in a crisis, businesses can rely on RAG to quickly identify the causes, and implementing solutions efficiently.
- Optimized hr: Instead of having a large team to handle information or answer the request from the client, the business can use the RAG to perform these tasks quickly, efficiently.
- Minimize errors: System access, auto-response eliminates the errors caused by humans, thereby avoiding the costs incurred are not necessary.
5. 5 outstanding application of Retrieval Augmented Generation
Applying RAG not only bring flexibility and accuracy in processing information, but also open up many innovative solutions for the business operations.
Here are 5 apps highlights of RAG to help optimize workflow and increase operational efficiency.
5.1 System faq advanced
System faq based on the RAG, not merely answer questions, but also give the accurate feedback, consistent with the context. Thanks to the ability to integrate data access and automatic feedback system, this is becoming an indispensable tool in an enterprise environment.
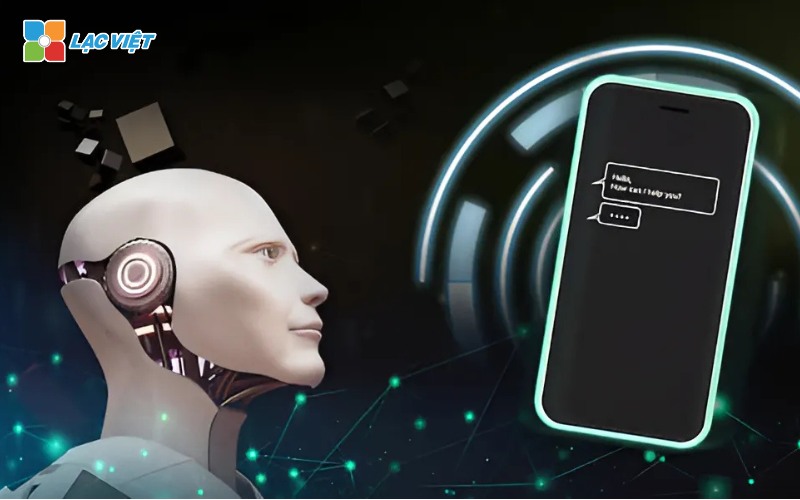
How to RAG operated in the faq:
When users take out of the question, Retrieval Augmented Generation, retrieving information from the database that are related and combined with context query. For example, if new employees need to learn the internal processes, the system will provide material, suitable explanation, help reduce the search time information.
5.2 Access to information quickly
One of the strong points of RAG is capable of handling, provide information from a huge amount of data in just a snap. This is especially useful for large enterprises, where the data is stored in the warehouse complex.
Such in the health sector, the system can support, doctor, access medical records, treatment history in just a few seconds, help improve the efficiency of health care.
5.3 Improve the conversation with the Chatbot
Other with chatbot typically, the chatbot integrated Retrieval Augmented Generation have the ability to provide feedback, carries in-depth, rich in context, even beyond the ability of the chatbot traditional. RAG not only based on the scenario programming available but also use real data to answer the query. For example, in the field of ecommerce chatbot can provide detailed information about the products accompanied by reviews from previous customers.
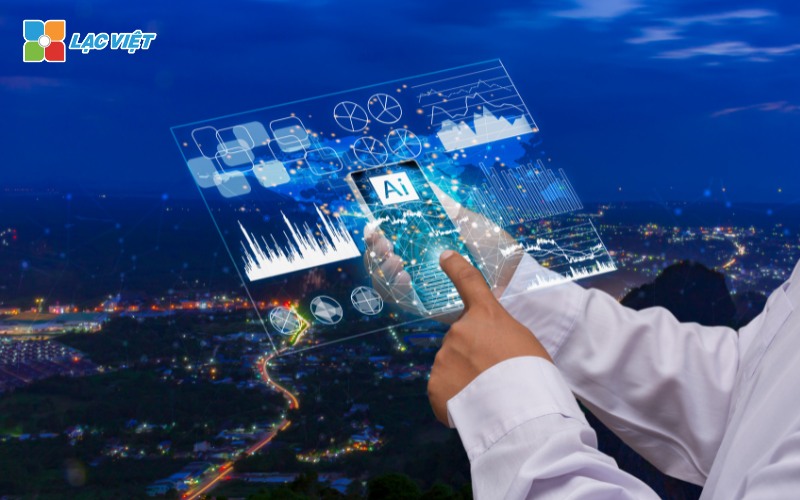
Lac Viet Chatbot AI Assistant is a tools app using artificial intelligence to support business optimization tasks in operations management. At the same time, Vietnam Chatbot AI Assistant also has the ability to integrate into the management software, the other to synthetic data, research, analysis, assessments, predict overview.
Virtual assistant answered 24/7 information internal business
Lac Viet Chatbot AI Assistant support 24/7 to answer any policies/mode Financial accounting with any information, Questions & Answers thanks to the integrated platform ChatGPT, Gemini ...
- Answer all of the information from the Document with all contexts, instead of searching manually.
- Automatic synthesis of information to the user after a search in the Source data.
- Automatic summary when the question – and-answer on a document file in the archives Of quick test – test full, Read fast – Read enough to help comply with and carry out the process.
Support operational accounting
- Chatbot AI to answer any queries in real time right in functional statistics report help Leadership decisions quickly, reducing the time to explain the report.
- Tracking and analysis of financial indicators, warning fluctuations instant help businesses manage risks proactively.
- Automation scheduling, Email reminders when to term liabilities – payments, increased experience with Customers/suppliers.
- Financial forecast accurately with AI analysis, historical data, predict trends, help plan financial efficiency.
Optimal process lookup – approved
- Integrated in the management system documents, the register number, to help answer any queries in real time right in the workspace.
- The analysis of data, business optimization, management accounting, to digitize processes approved.
Do you know businesses are spending a lot of money to pay for staff looking for information?
- Of 1.8 hours per day employees spend out to search and collect information, the equivalent of 9.3 hours per week
- Business loss 500 hours per year for employees to perform searches for information for work
- 63% leadership said the sharing of knowledge and information internal trouble, reduce the productivity of the business
Lac Viet Chatbot AI assistant – Freeing up personnel to focus on creative work
- Virtual assistant process – approved LV Chatbot AI for Workflow: Access quick information, content summary, revise errors on file the signed
- Virtual assistant accountant LV Chatbot AI assistant for Finance: remove input crafts, bring the data to the correct input, automatically prompt-term LIABILITIES – PAYMENTS, cash flow forecasting, warning of financial risks
- Virtual assistant customer care LV CareBot AI assistant: Integrated Chat on multi-platform, feedback and customer requests quickly, consulting, flexible, not being constrained by fixed script
- Virtual assistant hr LV Chatbot AI for HXM: save 70% time for HR and leadership, extract the entire database of candidates any file format, faq auto welfare policies, rules, regulations 24/7, statistical, personnel, resources, business in few seconds.
CONTACT INFORMATION:
- Lac Viet Informatics Joint Stock Company
- Hotline: 0901 555 063 | (+84.28) 3842 3333
- Email: info@lacviet.vn – Website: https://lacviet.vn
- Headquarters: 23 Nguyen Thi Huynh, P. 8, Q. Phu Nhuan, ho chi minh CITY. Ho Chi Minh
5.4 Create and summary of contents exactly
In the information age, the data processing huge volume of the information is succinct, easy to understand is a challenge. RAG business support, generate reports, summary of content quickly, saving time and cost.
For example in the field of journalism, reporters can use Retrieval Augmented Generation to summarize long articles into the main content, accessible to readers.
5.5 Support customer service
The ability to personalize customer feedback is the strong point of the RAG, to help businesses build trust, increase the satisfaction level of customers.
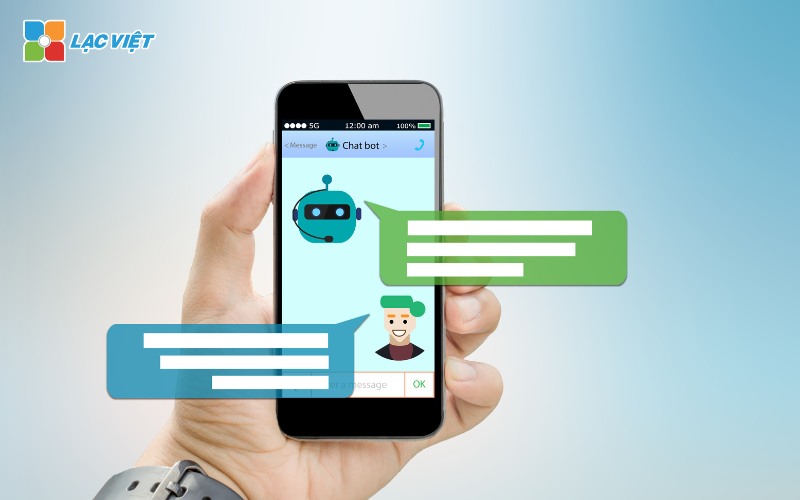
RAG helps chatbot not only understand but also feedback according to personal style, based on purchase history, behavior, access or emotional state of the customer. In addition, the system also supports expert sales consultants in the product description, the proposed solution is based on the individual needs of each customer.
6. Process deployment RAG for business
The application of Retrieval Augmented Generation (RAG) into business operations not only in technology but also as an overall strategy, which should be done methodically and scientifically. To ensure the system RAG bring practical value, businesses need to comply with a deployment process clearly and effectively.
Here are 5 basic steps to help businesses optimize the integrated RAG into the internal system.
Step 1: Determine the needs and goals of the business
To deploy successful Retrieval Augmented Generationthe first step is to clearly define your needs and goals of the business. This helps to ensure that the solution RAG matching strategy, bringing optimal efficiency.
Analysis of specific needs:
- Enterprises need to improve the customer experience through chatbot?
- Need support staff lookup internal information quickly?
- Need to increase the accuracy for the report automatically?
- Need to enhance the ability to query data in real time?
Define clear goals:
- Discount 30% response rate deviations from chatbot customer support.
- Increase the search speed, internal documents up 50%.
- Ensure the AI system provides information from the data source is updated.
Step 2: Prepare data base and model WHO
The deployment RAG require business fully prepared input data and models WHO available to integrate effective.
- Determine the type of data: Text data from internal documents, technical manuals, FAQs, information products/services, customer data,...
- Standardize – cleaning data: Remove outdated data or incorrect. Ensure data clearly structured to WHO can access easily.
- Prepare model WHO is there: Specify the language models are used (for example, GPT-3, GPT-4, or the custom model). Evaluate the possibility of integration of models with features to retrieve data.
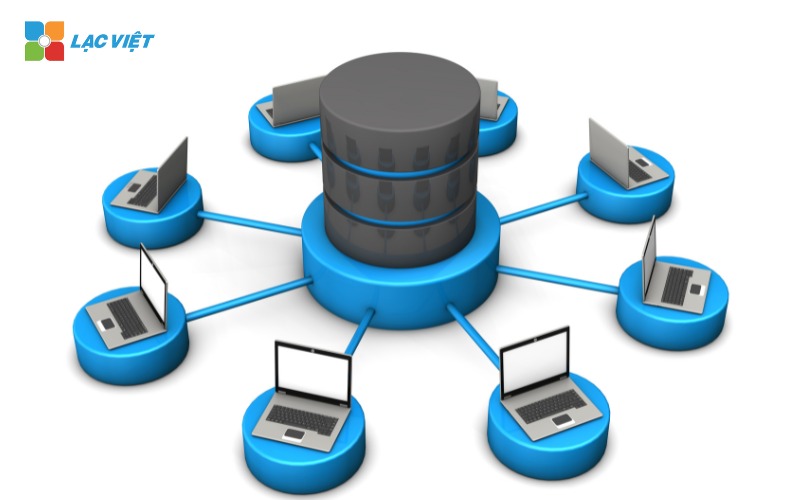
Step 3: integrate RAG into the current system
When prepared the database and model WHO, the next step is integrated Retrieval Augmented Generation into the system of the business.
- Select integrated solution suitable:
- On-premise (on-site): Suitable for businesses that need high data security.
- Cloud-based (cloud): Suitable for business needs the ability to expand quickly.
- Connect database with the pattern AI: Use the API or framework support (for example: LangChain, Haystack) to connect between the data and model WHO.
- Set the access and content: Specify the check points to ensure information is accurate traceability before model born answers.
- Integrated with the current system: Integrate with CRM systems to chatbot RAG providing customer information accurately. Connection with the management system documentation to employee search internal information easily.
The purpose of this step is the help system of the RAG is connected, works in sync with the existing tools of business.
Step 4: test and evaluate the effectiveness
After integration, it should proceed test thoroughly to ensure RAG operate effectively to meet goals.
- Functional testing: Ensure model to retrieve data accurately and provide suitable answers.
- Test performance: Rated speed feedback, the ability to handle as many simultaneous queries.
- Test security: System test to ensure no leaking sensitive data.
- Evaluation results based on KPIs: Measure response rate, accuracy, speed search, and the level of satisfaction of users.
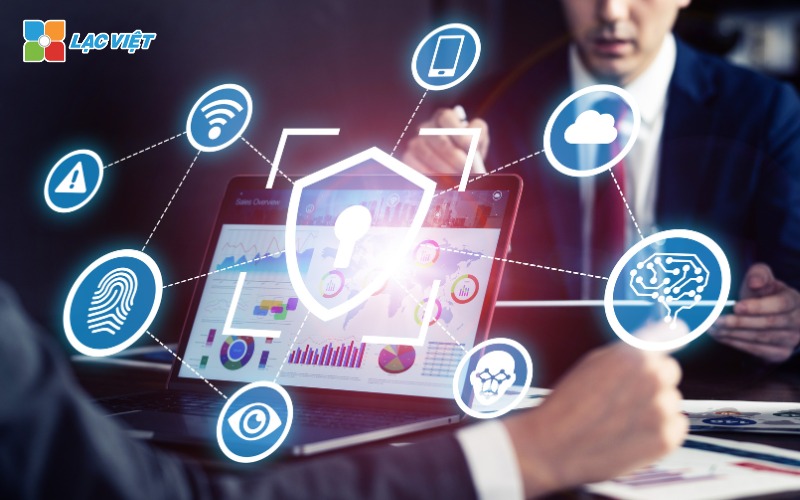
Step 5: optimize and scale
After testing and evaluating success, business conduct optimized scaling, deployment, Retrieval Augmented Generation.
- System optimization: Improving models to enhance the accuracy and speed feedback. At the same time, adjust the database to update with new information constantly.
- Coaching model more data peculiarities: Customize the model to better fit the language needs of the business.
- Scaling applied: Integrated RAG into many different parts, such as parts, customer support, legal department (research and general legal regulations), The technical department (search assistance, guidance on repair, maintenance),...
- Periodic review: Continuous monitoring, system improvements to meet business needs change.
Thanks to this step, system RAG operate stable, efficient and is applicable over a wide area in business.
7. Challenges when applying RAG and solutions for business
Deployment Retrieval Augmented Generation brings many advantages for business, but also comes with no less challenging. Identifying and addressing these obstacles is the key to optimize the efficiency of the system RAG.
7.1 Data access to heterogeneous
In the vast majority of business data is often stored in many different formats such as PDF, Word, Excel, or even no data structure from the email and notes. This makes the extracted information to the coaching model Retrieval Augmented Generation (RAG) much difficulty. Data heterogeneity can reduce the accuracy of information retrieval, which leads to results not consistently affect the operational efficiency of the chatbot.
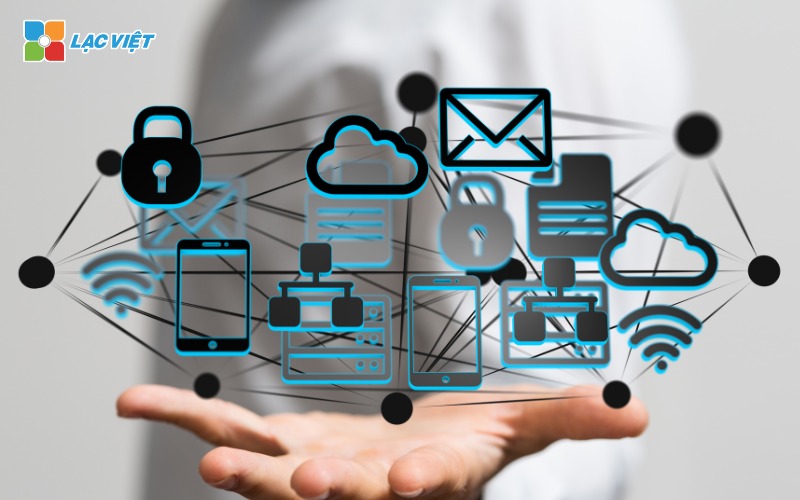
Solution fix:
Business needs planning and standardize data before deploying RAG. This process includes:
- Sort, classify data according to each group of functions or departments.
- Data conversion to the unified format such as JSON or XML for easy handling.
- Use OCR technology combined with AI-Data Extraction in order to automatically extract information from the document does not structure.
- Coaching and ongoing updates to chatbot properly understand the context, improving the ability to retrieve information.
Solution Server AI của Lạc Việt Ứng có khả năng tự động nhận diện, thu thập thông tin từ văn bản không có cấu trúc. Kiểm soát hoàn toàn dữ liệu đưa vào AI, dễ dàng huấn luyện AI phù hợp với nhu cầu cụ thể, không phụ thuộc vào dịch vụ của bên thứ ba.
According to the survey 2023 by IDC, more 95% the business world has started to convert numbers with different steps from learn, study, to start the deployment and implementation. Is step premise of the transition of document digitization – the opportunity to move his business in Vietnam when the state put in place policies to support businesses during the digitized.
Lac Viet – the first successful deployment service digitization OCR built-in AI for business
- OCR technology character recognition advanced, has the ability to convert images and scan documents into digital text with high accuracy, supports multi-languages, including English accented.
- Automatically recognizes, collects the information from the document does not have the structure (such as invoices, contracts, reports).
- Automatic sorting, converting these documents into a format that data (such as JSON), ready for storage, retrieval or integration into other systems.
- Integrated features translation auto for digitized documents, support more than 87 languages. Supported by LLM, features ensure the quality of translation retains context and meaning, especially useful for documents or international businesses with multi-national operations.
- Integrated chatbot AI smart allows queries to search data from the internal documents quickly.
SEE THE DETAILED FEATURES OF THE NUMERICAL SOLUTION HERE.
CONTACT INFORMATION:
- Lac Viet Informatics Joint Stock Company
- Hotline: 0901 555 063 | (+84.28) 3842 3333
- Email: info@lacviet.vn – Website: https://lacviet.vn
- Headquarters: 23 Nguyen Thi Huynh, P. 8, Q. Phu Nhuan, ho chi minh CITY. Ho Chi Minh
7.2 Cost of initial deployment
Applying RAG on chatbot WHO require the cost of the initial investment is quite big for infrastructure, technology, personnel expertise, systems integration. This can be big hurdle for the small and medium enterprises, which have limited budget for the project number conversion.
Solution fix:
- Select implementation partner prestigious as Lac Viet Computingsupply unit LV Chatbot AI Assistant with many services flexibility, including the form of rent, or buy software.
- Integrated gradually phased to allocate the budget reasonable, reduce the financial pressure.
- Leverage the infrastructure availableintegrated with systems such as CRM, ERP to optimize investment costs.
- Support free consultation and demo from our team of experts to ensure solutions fit with the real needs of the business.
Retrieval Augmented Generation not only is an advanced technology, but also as a powerful tool to help businesses optimize the ability to access information, improve customer experience, increase efficiency in internal operations. With the ability to mix between the search data and model language (LLM), RAG brings the groundbreaking solution, from system faq advanced to automate content, support customer service. Not to be left behind, the businesses need to actively capture al, deployment of modern technologies such as RAG, open up development opportunities durable advice, improve operational efficiency in the future.
CONTACT INFORMATION:
- Lac Viet Informatics Joint Stock Company
- Hotline: 0901 555 063 | (+84.28) 3842 3333
- Email: info@lacviet.vn – Website: https://lacviet.vn
- Headquarters: 23 Nguyen Thi Huynh, P. 8, Q. Phu Nhuan, ho chi minh CITY. Ho Chi Minh